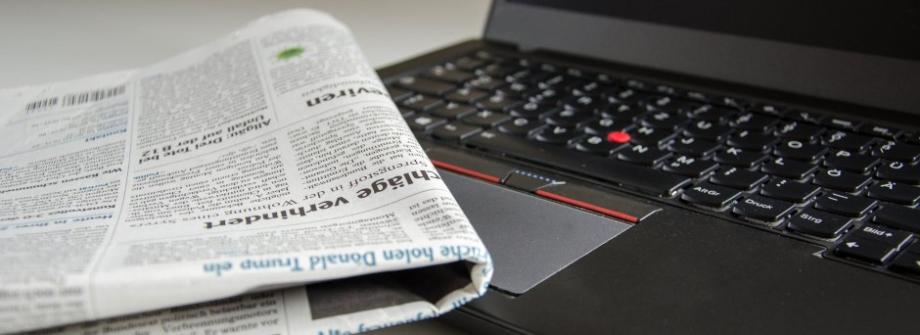
Meral Beksac* comments on the publication entitled “Genomic Classification and Individualized Prognosis in Multiple Myeloma” published in the Journal of Clinical Oncology.
* Meral Beksac, MD, Istinye University Ankara Liv Hospital Haematology and Stem Cell Transplantation Unit.
Genomic Classification and Individualized Prognosis in Multiple Myeloma
F Maura et al J Clin Oncol 9 Jan 2024 DOI https://doi.org/10.1200/JCO.23.01277
Autologous Stem Cell Transplantation (ASCT) has been the mainstay of myeloma treatment for more than four decades. Recently, new and highly successful induction regimens have initiated a discussion on “whether all myeloma transplant eligible patients should receive ASCT. We have known for a long time that Multiple Myeloma is not a single disease but instead covers multiple subgroups with a heterogenous spectrum of clinical, molecular and prognostic features. During the last two decades, many groups have tried to identify a molecular classification of myeloma(1,2). Among many, Sky92 has been validated in clinical trials and advanced to commercial level. Recently, the Heidelberg group have reported the complimentary role of combining Sky92 with FISH to define a double high risk group(3). In search for a more robust and validated prognostic risk model, a group of investigators led by Maura et al from University of Miami, have developed an Artificial Intelligence assisted risk classifier published in J Clinical Oncology(4).
In this publication, the investigators have developed a classifier to predict individualized risk for newly diagnosed myeloma (IRMMa). The study consists of two parts: the first is based on data from 1933 patients from multiple collaborators including CoMMpass and myeloma groups to develop a new genomic classification of newly diagnosed Multiple Myeloma(NDMM): 12 distinct biological subgroups of NDMM have been defined (https://github.com/UM-Myeloma-Genomics/GCP_MM). The clinical outcome among 12 biological subgroups according to treatment(ASCT +/- or maintenance +/- ) is as follows demonstrating a shift towards better outcome across biological subgroups with ASCT + maintenance(MCT).
In the second part, an individualized prediction model for clinical outcomes, that integrate genomic, clinical, treatment, and demographic features, was built. This innovative approach using deep learning and integration of genomic features plus 32 different treatment combinations (mostly proteasome inhibitors and IMiDs) led to the development of IRMMa. IRMMa aims to be a better genomics-based classification compared to current FISH- and gene expression (GEP) based classifications. Authors have integrated copy number variation , single-nucleotide variation and RNA sequencing data to reach this goal. The integration of CNV and mutations have been found to have an impact on patient clinical outcomes: high APOBEC and the presence of chromothriptic CNV signatures were strongly associated with poor outcomes. In addition, patients with bi-allelic loss of TP53, DNMT3A, or RB1 were found to have poor survival in this study, to confirm earlier reports. To correct for time dependent variables, including high-dose melphalan followed by ASCT, and maintenance therapy, a multistate model was designed. 20 genomics features emerged as important in predicting patients who progressed during the induction (i.e., refractory) and significantly boosted accuracy for overall survival. Among clinical features evaluated, age and ISS were the most important for model accuracy.
The IRMMa model accuracy was significantly higher than all comparator prognostic models, with a c-index for OS of 0.726, compared with International Staging System (ISS; 0.61), revised-ISS (0.572), and R2-ISS (0.625).
The current model allocated patients into six main clusters:
In cluster 1(n= 554), the intensive combination of ASCT plus maintenance/continuous treatment was effective in converting unfavorable outcomes into favorable ones.
In cluster 2 (n = 476), patients had a high treatment variance with significant benefit from receiving ASCT and a relatively small advantage in receiving maintenance/continuous treatment .
In cluster 3(n = 717), patients were usually age younger than 65 years with low ISS and low genomic complexity. In line with this presentation, any consolidation strategy provided an advantage, with no significant difference between ASCT and other maintenance/continuous treatments.
The other three groups included a smaller number of patients, with cluster 4 (n = 13) associated with favorable outcomes independent of the postinduction strategy. Cluster 5 (n= 155) was enriched for patients with high-risk genomic and clinical features, and poor outcomes, partially improved by ASCT. Clusters 6 (n = 18) included a small number of patients with aggressive clinical and genomic features and limited treatment variance.
The accuracy and superiority of IRMMa compared to other models was validated on an external set of 256 patients enrolled in a clinical trial. GMMG-HD6 (NCT02495922) aims to investigate the impact of addition of the anti-SLAMF7 moAb elotuzumab to lenalidomide / bortezomib / dexamethasone (RVd) in induction and consolidation therapy as well as to lenalidomide maintenance treatment in transplant-eligible NDMM. This trial was not able to show any benefit of Elotuzumab either during induction or consolidation(5). Patients enrolled in the GMMGHD6 were mostly assigned to clusters 1 and 3, suggesting that a fraction of patients might have had favorable outcome even without ASCT.
IRMMa is currently accessible for free allowing for estimations of individual patient risk and treatment variance: https://irmma-risk-calculator.miami.edu. Currently IRMMa is a research tool and can only be used for research purposes. In case of further validation among with patients treated with anti-CD38 MoAb accuracy of the model can be improved for more uptodate treatments allowing contemporary optimized individualized strategies.
References:
- Maura F, Bergsagel L. Molecular pathogenesis of Multiple Myeloma. Clinical implications Hematol Oncol Clin N Am - (2023) doi.10.1016/j.hoc.2023.12.010
- Aktas Samur et al. Deciphering the chronology of copy number alterations in Multiple Myeloma. Blood Cancer Journal ( 2019) 9:39. Doi.10.1038/s41408-019-0199-3
- Kuiper R et al Prognostic and predictive performance of R-ISS with SKY92 in older patients with multiple myeloma: the HOVON-87/NMSG-18 trial.doi.10.1182/bloodadvances.2020002838
- Francesco Maura et al Genomic Classification and Individualized Prognosis in Multiple Myeloma J Clin Oncol 9 Jan 2024 DOI https://doi.org/10.1200/JCO.23.01277
- Goldschmidt H et al Elotuzumab in Combination with Lenalidomide, Bortezomib, Dexamethasone and Autologous Transplantation for Newly-Diagnosed Multiple Myeloma: Results from the Randomized Phase III GMMG-HD6 Trial //doi.org/10.1182/blood-2021-147323